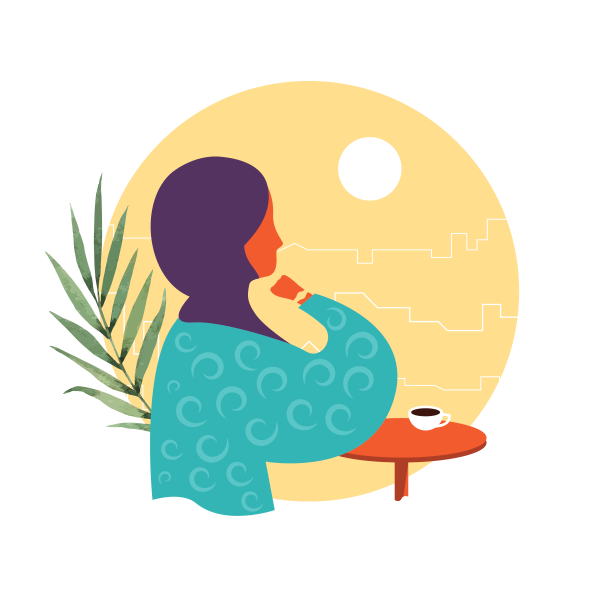
Thank you! You should receive an email asking you to confirm your subscription.
Oops! Something went wrong while submitting the form.
October 31, 2023
This article was written by Dr. Alexandra Pittman, Founder & CEO of ImpactMapper, and originally published as part of a series of engaging posts as part of International Data Week 2023 for Ryan Ginard's futurephilanthropy.blog
By 2030, it is estimated that at least $12 trillion market value will be unlocked through progressing on the SDGs around the world. Data on social impact and SDGs is growing at unprecedented rates.
As a sector, we are sitting on mountains of unanalyzed data, the majority of which is text. We must unlock insights hidden in text data to be successful and drive funding to the most impactful solutions. But how do we do that and at scale in secure ways?
The biggest barrier to understanding what is working in the philanthropic, investment and corporate sectors is the inability to process massive amounts of text data in an efficient and meaningful way. To start you need to create structured database for analysis. Identify key documents and excel files and pull out key sections of text, impact metrics, financials, and structure them into one master database to be analyzed. For example in a UNDP evaluation on gender equality and women’s empowerment and gender mainstreaming that I was the methodologist on, we created a master database combining all of the results from the internal UNDP results management database that had been collected over a five-year strategic planning period and also including outcomes and stories that we collected from field visits in different countries. Merging these two datasets and aligning the data with the UNDP country office that the results were connected to allow for a more robust blend of qualitative and quantitative data tied to the country as well as funding streams for gender equality. Creating comprehensive social impact databases and ensuring they are clean and ready for analysis is one of the most important and often overlooked steps in data analysis. Next we were ready to analyze the data.
Within this text data, incredible social insights and trends reside if you apply systematic qualitative data analysis and coding techniques. You can create codes for example, that help you to surface the process and outcomes of a social change progress to understand better what is working and what is not, e.g., positive and negative contextual conditions, strategies that are working or not, outcomes being achieved or thwarted, and lessons learned or recommendations.
There are two types of coding strategies that you can apply to text: inductive coding and deductive coding strategies. Inductive coding is when you create codes or identify thematic trends from the text data itself, so you are surfacing and aggregating trends from the text up. This is useful if you want a comprehensive picture of all of the outcomes that grantees are contributing to. We do this type of work with our clients at ImpactMapper when a foundation or organization is at a stage where they want to understand all the results and challenges grantees are facing. It is often done at a time when a new strategic planning or theory of change process is beginning so they have rich and comprehensive insights to guide the strategic reflection process.
Deductive coding is conducted when you already have established a taxonomy or coding structure. You then look for these codes and themes in the text, code the relevant text, and then aggregate trends. Most of the clients we work with use a deductive coding strategy because they already have a theory of change or strategic plans with indicators they want to track in a dataset. For more on applying qualitative coding techniques to philanthropic and nonprofit data, see this manual I wrote for foundations.
Once you have your dataset, you are ready to analyze it. It is most likely that you will want to use software to do so, like ImpactMapper as it makes coding, aggregating, and charting the trends easy. There are also other tools that many people use such as NVIVO or Atlas.TI (for only qualitative data analysis), Dedoose, or even Excel at the most basic level. But ImpactMapper is tailored specifically for the analysis needs of the philanthropic and impact investment spaces, allowing for financial, quantitative metrics, and qualitative data to be analyzed together.
To give an example of the power of coding qualitative data, I will continue with the UNDP evaluation example above. In this case, I developed a coding framework to measure the quality of the gender results gathered, called the Gender Results Effectiveness Scale (GRES). The scale aimed to assess the level of gender transformation and to assess the extent to which UNDP had achieved the objectives stated in their 5-year Strategic Plan and Gender Strategy of moving forward gender responsive and gender transformative outcomes. Too often in gender equality work, people treat all results the same, but that is not the case. The GRES allows groups and evaluators to speak in more granularity about results; for example, is the result primarily focused on counting the number of men or women (gender targeted), or is it truly moving to shift power and gendered social norms in communities or institutions (gender transformative)? The GRES gave operational definitions for gender blind, gender negative, gender-targeted, gender-responsive, and gender transformative results. Our team then coded each result in the database we created according to these five GRES areas and aggregated the results across the four strategic plan areas: Governance, Poverty Reduction, Crisis and Recovery, and Energy and Environment. The results showed that the majority of UNDP’s work in that 5-year period was gender targeted except for the Governance work, which was gender-responsive. This data was not on track with the strategic plan goals and allowed the Independent Evaluation Office at UNDP to start embedding a more directed and specific strategy and developed toolkits and trainings to support gender-responsive design and evaluations, thus strengthening how the agency responded and prioritized gender results in the future. This example illustrates how coding qualitative data surfaces critical trends for philanthropy and international development that can lead to better and more targeted interventions and better allocation of resources.
The future of qualitative data analysis lies in applying machine learning and artificial intelligence (AI) and automating the coding of text data.
Examining our relationship with social impact data and ensuring that social justice principles are embedded within software products is essential for the future of philanthropy. This is especially true given the rise of generative AI tools in our daily lives. Conversations around data security, privacy and equity, the use of predictive technologies, and artificial intelligence are becoming increasingly important in the social impact and philanthropic sector.
With the entrance of generative AI and Chat GPT, a wave of excitement has taken over the potential of applying AI to philanthropic data. While there is promise, one caution is that many of these models have been developed and trained on biased and discriminatory data that replicate biased assumptions in their prediction or recommendation models and systems. For example, just a few days ago researchers from Stanford Center for Research on Foundation Models (CRFM) Stanford Institute for Human-Centered Artificial Intelligence (HAI) released a study that tested ChatGPT and the more advanced GPT-4, both from OpenAI; Google’s Bard, and Anthropic’s Claude and found all four were giving false and biased medical information related to Black people based on stereotypes related to thickness of skin, kidney and lung function and a range of other issues. If this data was used to make medical or health decisions it could have disastrous consequences. And indeed we know that racially biased data like this has been used to make medical decisions in the past. For example, in 2019 an algorithm determining healthcare risk and the need for extra medical care in the US, was found to be racially biased, making recommendations for the extra care of white patients over black patients. This recommendation bias stemmed from the algorithm being trained on data that focused on previous patients’ healthcare spending. This data is a very poor indicator of actual healthcare needs in the US, given the privatized healthcare system, unequal distribution of financial wealth, and structural racism in the country1. The fact that biased medical recommendations in AI is still a problem after a lot of news coverage and many other similar cases is a significant issue. Philanthropic literacy in AI is key as is investing in tools that combat the biased algorithms that are the status quo today.
There is also a significant need for greater AI literacy in the philanthropic sector in terms of data security and privacy. Researchers at Stanford also just released this excellent report2 and created the Foundation Model Transparency Index ranking 10 open source and private AI companies3 on their transparency on multiple factors, including the model makeup in terms of data, labor, and computing power needed; model details, such as size, abilities, and risks; and use, distribution and geographic reach. The results of the research found that not one company scored more than 60% on the Index, underscoring the lack of transparency about the models, its uses, and risks.
Philanthropies need to examine the software tools they are currently using. It is important to understand privacy settings, who data is being shared with, what and how the data will be stored, who has access, if it could be shared back out to the public and in what form, if there are opt-out features to not share your data for training purposes, in addition to understanding the underlying AI models.
When choosing to use AI tools, it is also important to check the inclusiveness of the database the model was trained on. It is essential to ask how and what data was sourced, whose voices were included or not in training databases to develop models, how much data was biased or discriminatory, or coming from diverse perspectives, etc. ImpactMapper is at the forefront of doing this work equitably with its pilot funding from Malala Fund, which we are now scaling up with other donors. You can read more about the potential of inclusive and equitable AI for the sector, here Leveraging data science and AI to promote social justice, sustainability and equity. The focus on building technology with an equity focus includes reaching out to girls and women, social justice activists, LGBTQIA+, people of color, people living with disabilities, other minority groups, to ensure these voices lie at the base of training data that models and algorithms are built on, instead of relying on out of the box solutions from big corporations, many of which have been shown to have gender and racial bias built into their algorithms.
Girls, human rights, social justice, and climate activists’ voices are included in the training databases that we are building at ImpactMapper as part of our pilot work with Malala Fund, deepening equitable AI work in the philanthropic and impact sectors. Along with ImpactMapper, there also are many other exciting initiatives bringing equitable insights into the AI space from researchers and data scientists around the world, such as the A+ Alliance (Alliance for Inclusive Algorithms), AI for neurodiversity, the Data and Feminism Lab at MIT, Stanford Institute for Human-Centered Artificial Intelligence (HAI), Berkman Klein Center at Harvard, AI4SP.org, Alexander von Humboldt Institute for Internet and Society, and Ethics in AI at Oxford to name a few. These will be important places to watch and fund.
In sum, there is significant untapped potential in the vast amounts of text data related to social change and impact around the world. Now is the time to direct more resources to ensuring the software tools and models that are being built are equitable, transparent, and values-aligned in to truly accelerate social change, justice, and equity.